The Future of GRC: AI and Machine Learning in Risk Management
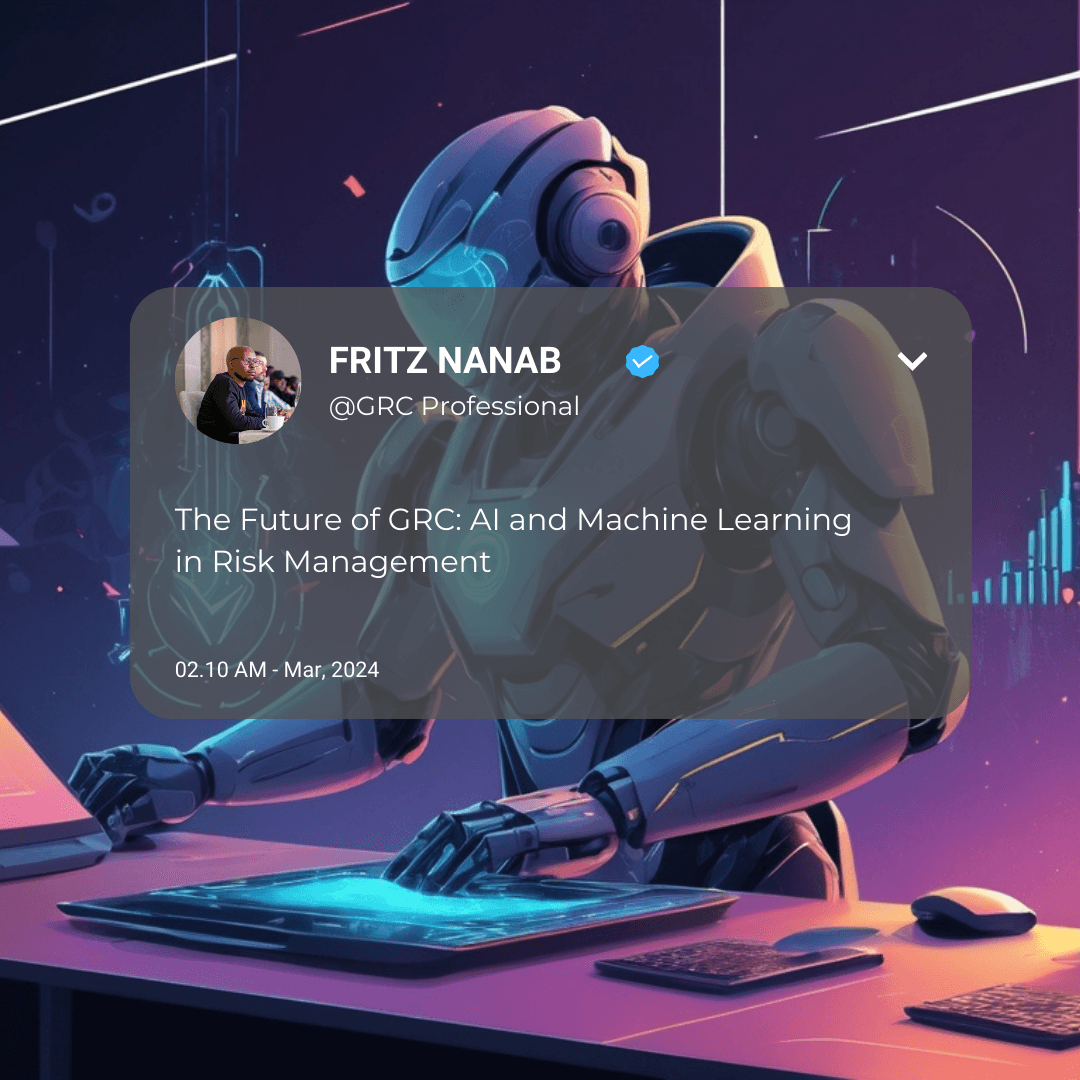
Introduction
In today's rapidly evolving business landscape, organizations face an increasingly complex web of risks. Traditional Governance, Risk, and Compliance (GRC) approaches are struggling to keep pace with the volume, velocity, and variety of data that must be analyzed to effectively manage risk. Enter Artificial Intelligence (AI) and Machine Learning (ML) – technologies that are poised to revolutionize the way we approach risk management within the GRC framework.
This blog post explores how AI and ML are shaping the future of GRC, particularly in the realm of risk management. We'll delve into the current challenges faced by risk management professionals, the transformative potential of AI and ML, and the practical applications that are already changing the game. By the end of this post, you'll have a clearer understanding of how these technologies can enhance your organization's risk management capabilities and what to consider when implementing AI-driven GRC solutions.
Current Challenges in Risk Management
Risk management professionals today face a myriad of challenges that traditional methods struggle to address effectively:
Data Overload: The sheer volume of data generated by modern businesses is overwhelming. From financial transactions and customer interactions to regulatory changes and market trends, risk managers must sift through an ever-growing mountain of information to identify potential threats.
Speed of Change: In our interconnected global economy, risks can emerge and evolve rapidly. By the time traditional risk assessment processes are complete, the landscape may have already shifted significantly.
Complexity of Risks: Modern risks are often interconnected and multifaceted, making it difficult to understand their full impact using conventional analysis methods.
Resource Constraints: Many organizations lack the human resources to thoroughly analyze all potential risks, leading to potential blind spots in their risk management strategies.
Regulatory Pressure: Increasing regulatory requirements across various industries demand more comprehensive and frequent risk assessments, straining existing resources and methodologies.
Bias and Human Error: Traditional risk assessment methods often rely heavily on human judgment, which can be subject to cognitive biases and errors, potentially leading to misjudged risk levels or overlooked threats.
These challenges highlight the limitations of conventional risk management approaches and underscore the need for more sophisticated, data-driven solutions. This is where AI and machine learning enter the picture, offering powerful tools to address these pain points and transform the way organizations approach risk management within their GRC frameworks..
The Role of AI and Machine Learning in GRC
Artificial Intelligence and Machine Learning are transforming the GRC landscape, offering powerful solutions to the challenges faced by risk management professionals. Here's how these technologies are reshaping the field:
Enhanced Data Processing: AI algorithms can process vast amounts of structured and unstructured data at speeds far beyond human capabilities. This allows for real-time risk assessment and more comprehensive analysis of potential threats.
Pattern Recognition: Machine learning models excel at identifying subtle patterns and correlations in data that might escape human notice. This capability enables early detection of emerging risks and more accurate prediction of potential issues.
Continuous Monitoring: AI-powered systems can provide ongoing, real-time monitoring of risk factors, allowing organizations to respond swiftly to changes in their risk profile.
Predictive Analytics: By analyzing historical data and current trends, AI can forecast potential future risks, enabling proactive risk management strategies.
Natural Language Processing (NLP): NLP capabilities allow AI systems to analyze text-based data sources, such as regulatory documents, news articles, and social media, to identify potential risks and compliance issues.
Automated Reporting: AI can generate detailed, data-driven risk reports, freeing up human resources for more strategic tasks and ensuring consistent, timely reporting.
Decision Support: While not replacing human judgment, AI can provide data-backed insights to support decision-making processes in risk management.
These capabilities address many of the challenges outlined in the previous section, offering a more robust, efficient, and proactive approach to risk management within the GRC framework. By leveraging AI and ML, organizations can enhance their ability to identify, assess, and mitigate risks in an increasingly complex business environment.
Key Applications of AI in Risk Management
AI and machine learning are being applied in various ways within risk management. Here are some key applications that are already making a significant impact:
Fraud Detection: AI algorithms can analyze transaction patterns, user behavior, and other data points to identify potential fraudulent activities in real-time, significantly improving detection rates and reducing false positives.
Cybersecurity: Machine learning models can detect anomalies in network traffic, user behavior, and system logs to identify potential security threats before they escalate into major incidents.
Regulatory Compliance: AI-powered tools can continuously scan regulatory changes, compare them against existing policies, and flag areas of potential non-compliance, helping organizations stay ahead of regulatory requirements.
Credit Risk Assessment: Machine learning models can analyze a wide range of data points to more accurately assess credit risk, leading to better lending decisions and reduced default rates.
Operational Risk Management: AI can analyze data from various sources (e.g., equipment sensors, maintenance logs, weather data) to predict potential operational failures and suggest preventive measures.
Market Risk Analysis: AI algorithms can process vast amounts of market data, news, and social media sentiment to provide more accurate and timely market risk assessments.
Supply Chain Risk Management: Machine learning can analyze supplier data, geopolitical events, and market trends to identify potential disruptions in the supply chain and suggest mitigation strategies.
Reputational Risk Monitoring: AI-powered sentiment analysis can monitor social media, news outlets, and customer feedback to detect potential reputational risks early.
Environmental, Social, and Governance (ESG) Risk Assessment: AI can analyze diverse data sources to evaluate an organization's performance on ESG factors and identify potential risks and opportunities.
These applications demonstrate the versatility and power of AI in addressing various aspects of risk management. By leveraging these technologies, organizations can achieve more comprehensive, accurate, and timely risk assessments, leading to better-informed decision-making and more effective risk mitigation strategies..
Benefits and Potential Drawbacks
While AI and machine learning offer significant advantages in risk management, it's important to consider both the benefits and potential drawbacks of implementing these technologies.
Benefits:
Improved Accuracy: AI can process and analyze vast amounts of data with greater precision than traditional methods, reducing human error and bias.
Enhanced Efficiency: Automation of routine tasks frees up human resources for more strategic work, improving overall productivity.
Real-time Insights: AI enables continuous monitoring and analysis, providing up-to-the-minute risk assessments.
Predictive Capabilities: Machine learning models can identify emerging risks and trends, allowing for proactive risk management.
Scalability: AI systems can easily scale to handle increasing data volumes and complexity without a proportional increase in resources.
Consistent Decision-making: AI applies consistent criteria across all assessments, ensuring uniformity in risk evaluation processes.
Potential Drawbacks:
Data Quality Dependency: The effectiveness of AI models relies heavily on the quality and completeness of input data. Poor data can lead to inaccurate results.
Lack of Contextual Understanding: AI may miss nuanced contextual factors that human experts would consider in risk assessment.
Transparency Concerns: Some AI models, particularly deep learning systems, can be "black boxes," making it difficult to explain their decision-making processes.
Implementation Costs: Initial investment in AI technologies and the necessary infrastructure can be substantial.
Skills Gap: Organizations may face challenges in finding or developing talent with the necessary skills to implement and manage AI systems effectively.
Overreliance on Technology: There's a risk of becoming too dependent on AI systems, potentially dulling human expertise and intuition over time.
Ethical Considerations: AI systems may inadvertently perpetuate biases present in historical data or raise privacy concerns in data collection and analysis.
Understanding these benefits and drawbacks is crucial for organizations considering the implementation of AI in their risk management processes. In the next section, we'll discuss key considerations for successfully integrating AI into GRC frameworks.
Implementation Considerations
Implementing AI and machine learning in risk management requires careful planning and consideration. Here are key factors organizations should address:
Strategic Alignment: Ensure that AI implementation aligns with your organization's overall GRC strategy and business objectives. Identify specific risk management processes that would benefit most from AI integration.
Data Strategy:
Assess the quality, availability, and accessibility of your data.
Implement robust data governance practices to ensure data integrity and compliance with privacy regulations.
Consider data integration challenges, especially if data is siloed across different systems.
Technology Infrastructure:
Evaluate your current IT infrastructure and determine if upgrades are necessary to support AI systems.
Consider cloud-based solutions for scalability and flexibility.
Skill Development:
Assess the current skill set of your team and identify gaps.
Invest in training programs to upskill existing staff or consider hiring specialists.
Foster collaboration between risk management professionals and data scientists.
Change Management:
Develop a change management strategy to address potential resistance to new technologies.
Communicate the benefits and limitations of AI to stakeholders clearly.
Ethical and Regulatory Compliance:
Ensure AI implementations comply with relevant regulations and ethical guidelines.
Implement mechanisms for monitoring and auditing AI systems to maintain transparency and accountability.
Start Small and Scale:
Begin with pilot projects to demonstrate value and gain organizational buy-in.
Use lessons learned from initial implementations to refine your approach before scaling up.
Vendor Selection:
If opting for third-party solutions, carefully evaluate vendors based on their expertise, track record, and alignment with your needs.
Ensure vendors can provide adequate support and ongoing maintenance.
Human-AI Collaboration:
Design processes that leverage the strengths of both AI and human experts.
Maintain human oversight and intervention capabilities in AI-driven processes.
Continuous Evaluation and Improvement:
Regularly assess the performance and impact of AI systems on your risk management processes.
Stay informed about advancements in AI and ML to ensure your implementation remains current and effective.
By carefully considering these factors, organizations can increase the likelihood of successful AI implementation in their risk management processes, realizing the benefits while mitigating potential drawbacks.
Conclusion
The integration of Artificial Intelligence and Machine Learning into Governance, Risk, and Compliance frameworks represents a significant leap forward in risk management capabilities. As we've explored throughout this post, these technologies offer powerful solutions to many of the challenges faced by modern risk management professionals.
By harnessing the power of AI and ML, organizations can process vast amounts of data, uncover hidden patterns, and generate real-time insights that were previously unattainable. From fraud detection and cybersecurity to regulatory compliance and operational risk management, AI is revolutionizing how we identify, assess, and mitigate risks.
However, it's crucial to approach AI implementation with a clear strategy and awareness of both its potential and limitations. Success requires careful consideration of data quality, infrastructure needs, skill development, and ethical implications. Organizations must strive for a balanced approach that combines the analytical power of AI with human expertise and judgment.
As we look to the future, it's clear that AI and ML will play an increasingly central role in GRC. Organizations that successfully integrate these technologies into their risk management processes will be better equipped to navigate the complex, fast-paced business environment of tomorrow. They will be more agile, more proactive, and better prepared to turn risk into opportunity.
The future of GRC is here, and it's powered by AI. Are you ready to embrace it?
This concludes my blog post on "The Future of GRC: AI and Machine Learning in Risk Management".
- Fritz Nanab